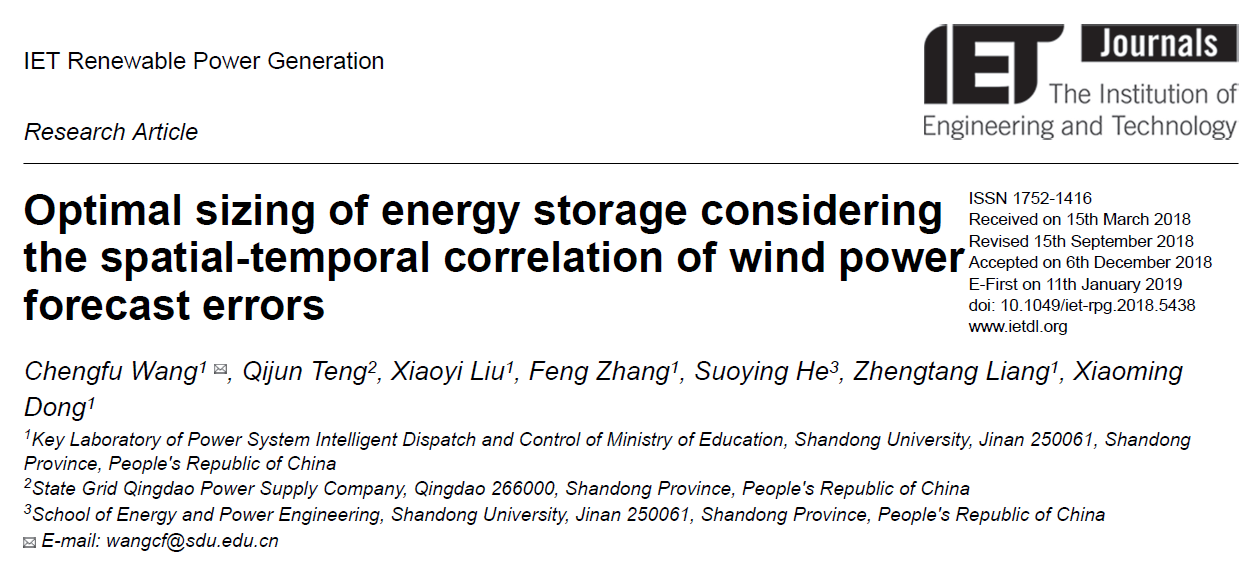
Introduction
Owing to increasingly severe energy resource and environmental problems, renewable energy, especially wind energy, has attracted considerable attention at the global scale and exhibited a rapidly increasing trend. However, due to the uncertainty and fluctuation of wind power generation, the large-scale integration of wind power introduces significant challenges to the operation of power system.
With the development of energy storage technology, the installation of energy storage system (ESS) can be treated as an effective method to mitigate the randomness and uncertainty of wind power generation. Nevertheless, how to size energy storage remains a significant challenge for the application of ESS since the high cost of energy storage device.
To determine the optimal size of ESS for wind farms, the balance between the economy of ESS sizing and the resulting performance of wind power fluctuation smoothing should be respected. Accordingly, concerning recent research, large amount of models have been built to economise the capacity or cost of ESS. Nevertheless, the scheme of power system operation and control are pre-deployed referring to the wind power prediction data, this prediction data is thus expected as closer to actual wind power output as possible. Therefore, the wind power forecasts or forecast errors have been considered during ESS sizing. A sizing method for ESS composed of different types of storage devices has been presented in to reduce the time-varying components of the forecast errors. An energy storage sizing method has been proposed in to effectively reduce the negative impact of short-term wind power forecast error. Baker et al. have proposed an ESS optimal sizing tool allowing for the forecast errors based on two-stage stochastic model predictive control. Overall, the studies above have verified the effectiveness of considering forecast error during ESS sizing and analysed the effect of error distribution on ESS sizing. However, the spatial-temporal correlation of forecast error has a remarkable impact on the temporality and accuracy of error distribution, and is ignored in previous research. Thus, this factor needs to be further considered to improve the accuracy of ESS sizing.
It has been verified that the correlation of wind power and its forecast error have a significant effect on the distribution of forecast error. Accordingly, a spatial-temporal correlation model for wind speed and wind power based on copula function has been proposed in. The proposed nonlinear modelling method can address the dependence accurately and can identify the zero dependence of wind data. Moreover, a simplified ESS model is applied to demonstrate the advantages and disadvantages of different correlation modelling approaches.
However, it may be more accurate and effective to directly use spatial-temporal dependencies of forecast errors than using dependencies of wind power or speed during ESS sizing. In order to capture the autocorrelation of day-ahead forecast error, a datafitted autoregressive process has been used to model forecast error. However, the proposed linear model has been not fit for the non-linear relationship of dataset, and the influences of spatial-temporal correlation of forecast errors on ESS sizing have not been mentioned either. In, the influence of forecast error autocorrelation to the sizing of ESS has been analysed in detail. In, the temporal correlation of forecast error based on Copula theory has been used to size the energy storage power and capacity. The works mentioned above have proved that the storage capacity would be misestimated if the autocorrelation of forecast errors is not taken into consideration. That is, it is necessary to consider the temporal correlation into rational energy storage sizing. However, there is also a strong spatial correlation between wind farms in the same regional power grid. These spatial correlations have not been considered in previous research of ESS. Therefore, it is necessary to further study the ESS sizing considering the spatial-temporal correlation of the forecast errors for multiple nearby wind farms.
On the other hand, the effect of different control strategies on ESS sizing is significant. An optimal control strategy for ESS has been built based on traditional feedback control method. An optimal sizing model for battery storage system has been proposed and the optimisation results of four controllers (simple, fuzzy, ANN and Adv. ANN) have been compared. A variable-interval reference signal optimization approach and a sizing method for BESS planning have been presented to smooth wind power and the fuzzy control-based scheme is used to decrease BESS capacity. The control strategies mentioned above are all based on known and deterministic information during the operation and control of ESS. However, the uncertain information such as forecast data should be considered during the operation of ESS to better deal with the wind power fluctuation.
Consequently, to reduce the effect of forecast error fluctuation, a novel optimal ESS sizing method is proposed considering the spatial-temporal correlation of forecast errors for multiple nearby wind farms. In order to improve the economic and operating benefits, the proposed method minimises the investment and operating costs of the ESS. In this method, a correlation model of multi-dimensional forecast errors based on Copula theory is built to guarantee the accuracy of correlation analysis. Meanwhile, an operation strategy including the trend of prediction and correlation of error is used to address optimal operation of ESS during energy storage sizing.
The remaining of this paper is organised as follows. The data source and a simple demonstration are described in Section 2. The spatial-temporal correlation of forecast error is modelled in Section 3. A new optimal energy storage sizing method is proposed in Section 4. The simulation results using different cases are demonstrated in Section 5. The conclusion is given in Section 6.